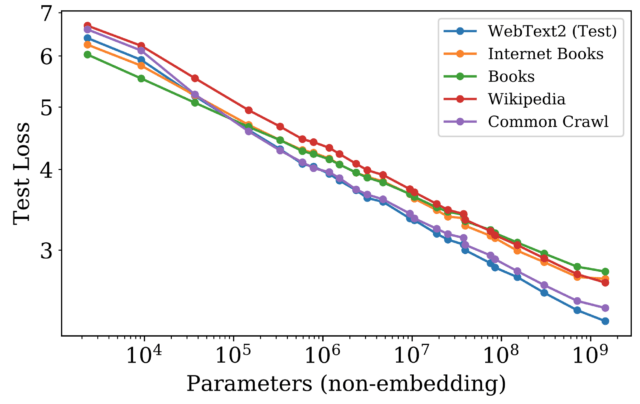
In the rapidly evolving field of artificial intelligence (AI), large language models (LLMs) like GPT-3 have been at the center of a fascinating debate: Do these models exhibit “emergent abilities” — capabilities not explicitly programmed into them but that arise as they scale up in size? This question has sparked extensive research, lively discussions, and a bit of controversy among experts. Here, we delve into the essence of emergent abilities, scrutinize the arguments on both sides, and explore the implications of this phenomenon for the future of AI.
Key Highlights:
- Definition and Examples of Emergent Abilities: Emergent abilities in LLMs refer to the phenomena where capabilities not present in smaller models become apparent in larger ones. This includes few-shot learning, where models apply learned patterns from a few examples to new, unseen tasks, and complex problem-solving without explicit training on those tasks.
- The Mirage of Emergence: Recent studies challenge the notion of emergent abilities, suggesting that what appears as emergent is often an artifact of the metrics used to measure performance. By changing from non-linear to linear metrics or increasing test data, purportedly emergent abilities become predictable improvements across model sizes. This perspective argues that the dramatic emergence of capabilities is more illusion than reality, influenced by the choice of evaluation methods
- The Role of Model Scale and Complexity: The transformer architecture, foundational to LLMs, enables parallel processing of large text volumes, contributing to the rapid scaling and complexity of models. As models like GPT-3 and Google’s PaLM have scaled up to hundreds of billions of parameters, they’ve shown remarkable abilities, sparking excitement and concern alike about AI’s potential.
- Debating the Reality of Emergent Abilities: Critics argue that LLMs, despite their sophisticated pattern recognition, do not truly understand or reason, but merely mimic comprehension based on statistical patterns in data. In contrast, proponents see emergent abilities as indicative of a complex, albeit non-human, form of learning and generalization.
- Implications and Future Directions: Whether real or illusory, emergent abilities in LLMs carry significant implications. They could enhance AI applications across various fields, from customer service to scientific research, by enabling more nuanced and sophisticated task performance. However, this potential also raises ethical and practical questions about AI’s use, fairness, and transparency.
The debate over emergent abilities in LLMs underscores the complexity of AI development and the challenges in understanding and measuring intelligence in machines. While recent critiques suggest that these abilities may be more about perception influenced by measurement techniques, the discussion highlights the importance of continued research. By better understanding the mechanisms behind LLMs’ capabilities, we can more effectively harness their potential, address ethical concerns, and shape the future of artificial intelligence.